The
Empathetic Internet Thanks to new
developments in data mining, computers are learning increasingly
detailed profiles of individual users. Erin Digitale
wonders how well she wants the web to get to know her. Illustrated
by Kacie Carter. 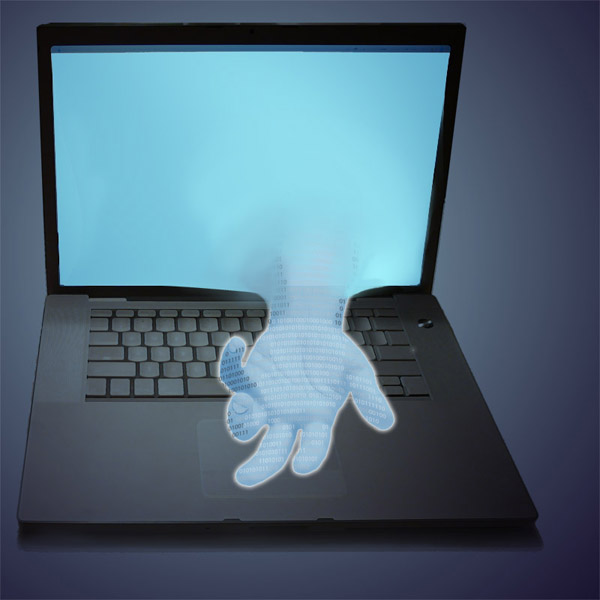 Illustration:
Kacie Carter There it
is, right on top of the recommended movie list in my familys Netflix
account: Mr.
Bean's Holiday. Ugh, I think.
I hate Mr. Bean. Has Netflix lost its cookies? No, but it is having trouble making good use of
its vast stockpile of user-generated movie ratings. Thats why the
popular web-based DVD rental company is offering a $1 million prize to improve its movie-recommendation
software. One contestant is UC Santa Cruz computer scientist Yi Zhang. Zhang and her
team study how computers learn from the data we generate when, for
example, we search the Internet or make online purchases. Were
trying to come up with algorithms to train machines to think like
human beings, says Anita Krishnakumar, a graduate student working
with Zhang. The Netflix contest is just
one part of Zhangs overall efforts. Her teams research, which
trains computers to give tailored responses to individual users,
will hone search-engine results and help businesses target online
advertising. The research will improve your computers ability not
just to fetch information from queries, but also to send you the
content you needunprompted. Ultimately, it aims to transform the
consumer portion of the Internet from an unwieldy behemoth with the
simple stimulus-response intelligence of a lizard to a well-trained,
useful beast with the ability to sort information rationally.
Most of Zhang's peers focus on isolated aspects
of this field, called data mining, such as exploiting online social
networks or helping computers understand language. Zhang says a
narrow focus would limit the usefulness of her research, so she
teaches computers to integrate many kinds of data. She makes novel
use of Bayesian analysis, a branch of
statistics that gives computers a logical architecture for sorting
and learning from new information. The human mindour headis a
unifying framework, and Im trying to build that, she says. But for all its potential benefits, data-mining
research like Zhangs also raises serious questions about computer
security and personal privacy. The fact that databases are learning
to talk to each other presents a distinct set of challenges for our
legal system, says Lauren Gelman, director of the Center for Internet and Society
at Stanford Law School. Whereas in the past, tracking an individual
through public records required digging through file drawers in
courthouses, today the same information is available with a few
keystrokes. Now, we have a very different privacy question, but
the laws have not changed, says Gelman. Measuring our longings Selective data filtering is nothing new. Mail-order
specialists at Sears and Montgomery Ward were knee-deep in customer
data by the turn of the 20th century. The New York-based Direct
Marketing Association was founded in 1917 to help make sense
of what direct-mail customers wanted. And clipping services, which
let organizations track media reports on their activities, have
been going strong for about 100 years. In
the old days, picking out trends in these records was a fuzzy
business. For instance, mail-order companies could track consumers
names, addresses, and some demographic tidbits such as gender or
age. But fine details on customers habits were inaccessible. A
turn-of-the-century clothing merchant had no way to figure out which
young ladies lingered covetously over his advertisement for an
Inflatable Elastic Bosomthe one that adjusted for all four stages
of a womans life (Miss, Debutante, Mother, and Dowager) and doubled
as a life preserver. Today, its different.
When I search inflatable bosom on Amazon.com, I get three books on
the history of womens fashions. Using my computers Internet Protocol
(IP) address, Amazon can track which books I click on, how long I
spend reading the product descriptions, whether I search the books
text, and what I decide to buy. The key
to such intense data collection is surplus computing power. Its
now easy to trail users all over the Internet in minute detail, and
cheap to store the maps of their trails. And because the torrents
of data seem like they might be useful someday, companies are
reluctant to ditch even the most mundane tidbits. Climbing Mt. Data But accumulating all that detail causes a new
problem: mountains of numbers. Its not just using the Internet
that leaves dense data trails. Scientists produce stacks of data
with high-powered telescopes that scan the skies, genetic investigations
that sort millions of DNA codings, and public-health studies that
track scores of people over many decades. On a personal scale, all
cellphone calls generate data. So do bank transactions, visits to
the doctor, and trips to the grocery store. Yi
Zhang is one of many researchers tunneling into this mountain. She
wants to enable computers to judge the data in a rational way, as
a person would. She knows this goal is a long way off. Meanwhile,
we are making things that are useful for people, she says.
Zhang works from a shiny green-glass building that
looks like a silicon computer chip dropped among the redwood trees
of UC Santa Cruz. Her office is sleek: modular desk, low couch,
few books. It seems too emptyuntil she opens a drawer and pulls
out stacks of CDs from Netflix, Microsoft, PubMed, and Reuters.
I like to play with this real data, she
says. Zhang didnt always see data as the
raw material for play. At first, sifting through numbers struck
her as simple, even boring. But as she tried to make computers
mimic human minds, the data increasingly drew her in: I finally
made what seems like a boring problem into a very interesting
problem. She enjoys slicing smart computing into manageable bites.
Right now, she says, although we cannot build a robot that can talk
intelligently, we can build something in the middle, like a search
engine. Search engines such as Google and
Yahoo already use basic data-mining techniques to tailor results
to the search histories of users. Suppose you enter spears into a
search engine, says Krishnakumar. The first time, it just recommends
everything, she says. Specialty websites about medieval spears, a
Wikipedia article about spears, Britneys latest anticstheyll all
pop up. But read about Britneys legal woes, then do the same search
a few more times, and the results change. After three or four days,
when you search again, you can see Britney Spears being ranked
higher up, Krishnakumar says. (For the
record, I had limited success with this experiment. Everything
came up Britney the first time. Of course, the results may
have been biased by the fact that my computer knows my secret
addiction to the website of People, which pantingly reports
Britneys every change of wig.) Still, todays
search engines can only get so close to you. Zhang wants to go
further, so her team is building recommendation systems. Search
engines make users hunt for new content; recommendation systems
suggest it automatically. Netflix, for instance, tracks the films
you rent, solicits your ratings, and considers which movies your
friends liked. It combines these factors to generate a profile
that drives the Films youll heart page. You can view it as a
proactive search engine, Zhang says. Recommendation
systems must be taught to question users intelligently, Zhang says,
in the same way new friends get to know each other. Rather than
asking a rote set of questions, the system has to learn actively
and adjust its questions on the fly. That means taking risks. So
instead of just suggesting content that matches your profile,
recommendation systems also add exploratory suggestions that push
the boundaries of your preferences. If you like one of these new
items, it opens a big box of treasures for you, Zhang says. Even
when you dislike a new item, the system still learns about you. If
you spend only a short time looking at a suggestion, or fail to
click web links from it, the system makes more inferences about
what you like. The systems goals parallel business goals, Zhang
notes: I want to make you happy now, and make you happy in the
future. Like humans getting to know
each other, recommendation systems need to be a bit cautious. The
information the machine has collected is very unreliable, noisy,
and biased, Zhang says. Some people try to cheat the machine, she
adds, such as those trying to promote their product. The system also must withstand the enormous volume
of data it encounters. The machine is living in a world thats
probably even more complicated than our human world, she says. Once
a real system is launched, it will face a lot of peoplemore people
than a human faces in his whole life, every single day. Rather
than using the brute-force approach of supplying more computing
power, Zhangs team is helping computers navigate the data onslaught
by showing them which calculations to focus on. Its similar to the
filtering that happens in the part of the human brain that unconsciously
sorts distracting noises from important sounds. Building the gears So computers running recommendation systems must
be adventurous, cautious, and adept with cascades of information.
How do they translate a bunch of ones and zeroes to these complex
traits? Creating the mathematical gears of
a recommendation system requires several steps. Zhangs team first
cleans its data by removing points that dont make sense, such as a
reported human age of 263 years. Then they
weed. The data have hundreds of different attributes, but it doesnt
make sense to use everything. A recommendation system gauging the
interests of users might pay attention to their ages and genders
but ignore their heights and eye colors. Once
the raw numbers are ready, Zhangs team must make an opening guess
at the mathematical equations that will best represent their data.
Graduate student Ethan Zhang (no relation) says the teams guess-and-check
approach parallels the way other scientists design experiments
without the Petri dishes or Erlenmeyer flasks. In the beginning,
there are no plans, just trial and error, he says. As the experiments
progress, the researchers check their new strategies against older
mathematical approaches to see if theyre improving on the old way
of doing things. Zhangs team builds its
guesses with the tools of Bayesian analysis, a branch of statistics
that combines old information with new data in a rational way.
Bayesian analysis translates starting beliefs about the data into
mathematical probabilities. The nascent recommendation system
begins by treating these probabilities as weights, then asks users
for additional weighted data: opinions. If you give a rating of
four for movie A, three for movie B, and five for movie C, you are
giving a weight to each of the movies, Krishnakumar says. The
weighted values add together to give a value for the entire equation.
With that value, you can come up with the next item to recommend
to the user, she says. A recommendation
system uses your feedback to weight categories of items. The system
constructs equations to represent your preferences for movie genres,
directors, items your friends liked, or items that got high ratings
from people the system thinks are similar to you. With each new
tidbit of data, the computer reruns the equations that describe
you. The calculations are much simpler than the wiring of your
brain, but the deluge of data helps the computer compensate for
this simplicity. With each new turn of the equations' cogs, the
system learns a little more about you. Many
computer scientists use groups of endlessly recalculated equations
to discern your preferences. Zhangs work stands out because it
extends the basic idea of reiterated calculations in both breadth
and depth. In addition to bringing together many types of data
under a unifying framework, Zhangs approach also teaches computers
to understand deeper nuances of a users preferences and needs. Its
not so hard to develop a model of what you might be interested in,
says Jamie Callan, a computer scientist
at Carnegie Mellon University who supervised Zhangs Ph.D. research.
But its much more difficult to depict the level of detail youre
looking for. This problem becomes significant
for recommendation systems that rate pieces of text. Are you reading
websites about heart disease because your grandfather just had a
heart attack, or because youre a cardiologist checking the newest
heart-attack treatments? Those two users, interested in the same
topic, need very different things from a recommendation system.
The system must gauge the level of detail in the material. Thats
a hard problem, Callan says. Many people have struggled with it.
Yi was one of the first people to do really good research. The way Zhang builds her recommendation systems
is unique, says Stephen Robertson, a
senior researcher at Microsoft who knows her work well. We tend
to have a black-box notion of users preferences, Robertson says.
Yi tried to disentangle that into different components. Robertson
says Zhangs approach is important because, rather than just solving
a single narrow search problem in an ad-hoc way, it takes a logical,
theory-based approach. To do this, Zhang
built her Bayesian analysis frameworks by consciously considering
the four main ways people learn: They use prior knowledge, ask good
questions, use context and feedback, and observe the behaviors of
others. She selected a different branch of Bayesian analysis to
represent each of these types of learning mathematically. Then,
she fitted the four analyses into a mathematical frame that shows
the computer how the various types of data connect to one another.
Getting to know a new friend, you care more about your big similarities
(she lives nearby, you enjoy the same activities) than trivial
differences (she drinks coffee, you dont). The math frame focuses
the computers attention on important similarities among users and
helps it ignore the trivia. Zhangs approach
is shedding light on the entire field of data mining, Robertson
says. Web search engines are limited in what they can do, he says.
Understanding those limitations is where we should be going.
Chasing down our privacy The increasing power of search engines has a down
side, say some observers. Data is the
pollution problem of the information age, says privacy expert Bruce
Schneier, who runs the computer security company BT Counterpane in Mountain View, California. The
question is whether information gets recycled after users disclose
it. The U.S. has no real laws protecting someone from doing
something with your information. The European
Unions privacy laws say information collected for one purpose cannot
be reused for another. Lack of similar U.S. laws is causing
problems. For example, social-networking site Facebook created an
uproar in late 2007 with a new feature that automatically broadcast
the online activities of users to their friends. Users voiced angry
complaints, saying it was too hard to opt out of the broadcast
feature. Facebook founder Mark Zuckerberg eventually apologized.
People need to be able to explicitly choose what they share, he
said in a blog message. Schneier agrees.
But he says most computer users dont understand what theyd have to
do to protect their information from being reused. Go ask your
mother how to opt out, he says. The rules are hard to find and the
technical ability isnt there. And without widespread awareness of
the problem, he says, there isnt adequate market demand for businesses
to invest in technology that would protect users privacy. Legislation
is the only way out, Schneier says. Lauren
Gelman, the Stanford law professor, worries that without enhanced
privacy laws, data mining will erode privacy to the point that it
dampens our culture. I take an approach to privacy that looks at
it as a community interest, a societal interest, she says. If we
live in a world where everything about what we do is being captured
and catalogued and becomes searchable, then well start thinking
twice about what we do. We need to leave holes in the system so
people can experiment. Gelmans law students already worry about
exchanging experimental ideas in class blogs. What if someone uses
those blogs 20 years down the road to derail a Supreme Court
nomination? As a society, its to our detriment if we start to
self-censor, she says. Computer scientists,
meanwhile, have mixed reactions to the question of how to protect
users privacy. Jamie Callan, Yi Zhangs Ph.D.
supervisor, agrees with Gelman and Schneier that the U.S. needs
new privacy laws. Its not instantly clear what those laws ought
to be, he says, but right now theres very little protection. As
work like Zhangs enables computers to integrate many kinds of data,
it will become increasingly possible to build incredibly detailed
models of what you do every minute of every day, Callan says. And
I think thats something the American public ought to be concerned
about. Zhang says shes no expert on privacy
law, but she sees promise in technical strategies for protecting
privacy. You can design the system so that, for non-public
information, you just ask users when they want to share it. Businesses
eventually will realize its in their economic interest to make users
happy by protecting their privacy, she says. Until that happens,
she thinks users should exercise caution about what they put on the
Internet. In the end, data-mining research
is double-edged. If researchers manage it correctly, this work
will turn the vast, unwieldy Internet into millions of individually
tailored internets which feed each of us information suited to our
idiosyncratic needs. At that point, Netflix will only recommend
Mr. Bean to those who truly appreciate his irritating wackiness.
But to benefit from the many internets of
the future, consumers must demand technologies that guard their
data or ask for laws prohibiting data reuse. Without these
protections, todays Bean-sized nuisance could morph into tomorrows
intrusive surveillance. Top
Biographies Erin Digitale B.Sc.
(biochemistry) University of British Columbia Ph.D. (nutrition)
University of California, Davis Health Research Communications
Award, Canadian Institutes of Health Research Internship:
Stanford Medical School news office In
grade three, I wrote a story about a girl hunting dinosaur fossils.
On the first day, she found a tiny fossil. On the second day, she
found a fossilized skull. And on the third day, she found an entire
Stegosaurus skeleton! My story, criticized
by an 8-year-old classmate as unrealistic, foreshadowed why Im
pursuing a career in writing instead of research. I dont want to
spend years digging in the same pile of dirt, as a good scientist
must. I want the chase, the climax, and the dnouementnow. Ive
earned two postsecondary degrees via esoterica such as watching
videos of wiggly enzymes, making rat-liver milkshakes, and lecturing
undergraduates on the capabilities of the human stomach. Now Im
eager to tell the stories of science beyond the academy walls.
. . . . . . . . . . . . . . . .
. . . . . . . . . . . . . . . . . . . . . .
. . . . . . . . . . . . . 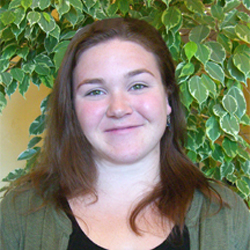
Kacie Carter B.A.
(environmental studies and legal studies) University of
California, Santa Cruz My childhood
on the California coast filled me with a love of exploring the
natural world through field science and artistic expression. I
found the perfect outlet for my lifelong passions in the Science
Illustration Program. Nothing is more beautiful or interesting
than nature, and the attempt to capture a tiny fraction of that
beauty brings me closer to understanding and being a part of that
whole. Science illustration is a synthesis of my passions; to me
it is an elegant combination of science, art, and environmental
education. In the brush stokes of watercolor, or in a simple pen
line, you can communicate the detail, form and wonder of the natural
world. It becomes immediately accessible, and provides a gateway
for understanding. Top |